Is your small business growing faster than you can keep up with? It might be time to grow your team, document key processes, and make sure you’re using the right technologies to scale up operations. In other words, your company might need business infrastructure. At Equilibria, Incorporated we specialize in building business infrastructure.
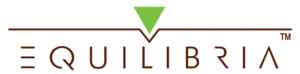
Equilibria is proud to sponsor this special season which features an AI audio conference to advance your knowledge of business infrastructure. We hope you enjoy this episode!
It’s Season 22 here on the Business Infrastructure podcast which features our first ever AI audio conference. I’m your host, Alicia Butler Pierre, and on this show, we share operational tips, strategies, and tactics to help you cure any back-office blues you might be experiencing.
We’re at the end of our conference and it features our closing fireside chat. If you’ve been wondering how you can build a custom AI tool to streamline your operations, then you’re in the right place!
Before we start, I must remind you of our disclaimer – the nature of AI technology is changing fast, so it’s possible some of the information in this episode might be dated since the original session. We encourage you to learn what you can, test it, and most importantly have fun! And now for our final fireside chat….
This is Episode 283 – Building Your Own AI Tools to Unleash Operational Efficiency with Tracy Hazzard.
Welcome everyone to our closing fireside chat with Tracy Hazzard, a woman that I’ve admired ever since I met her. She is the co-founder of Podetize, one of the largest podcast post-production companies in the world. She’s also a serial entrepreneur and has a long and impressive background in technology. Tracy has some exciting things to share with you – things that can potentially revolutionize the way you operate your business. But rather than me tell you, it’s best you hear it from her. Tracy, welcome! Please, feel free to introduce yourself.
Welcome to my presentation on customizing AI to optimize your business. My name is Tracy Hazzard. I’m the CEO and co-founder of Podetize. And we’re going to specifically talk about how to build data sets and feedback loops to customize and train your AI.
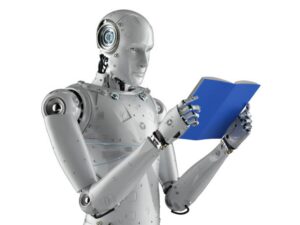
Tracy is very modest everyone. I’ll brag on her and Podetize a little bit. She’s personally conducted over 3,000 interviews and Podetize has produced over 1,000 shows with 50,000 episodes and counting for their clients. Wow! The last time you came onto the show Tracy you gave us a peek behind the curtain of Podetize. Turns out you all use ClickUp as an operating system but with all kinds of customizations to automate your workflow for an international team that spans several countries, right? How many countries is it?
Five countries, and we do still use ClickUp, and we have about 80 staff members that are in and out of the ClickUp process. It’s still going like gangbusters, but we customize it so much that it’s like unrecognizable as ClickUp. It just automates the production flow and keeps it trackable for us. So this is really why we could see overlaps and inefficiencies in our business really easily. And so right now, we’re actually in the process of integrating our audio and video team together because of AI tools. We have like more than 60% of our client base is now doing video as well, which is different than it has been in the last four years.
That makes sense, especially with the proliferation of shorts on platforms like TikTok, Instagram, and YouTube. Being that you’re a technologist at heart, Tracy, I think it’s safe to say that Podetize is an early adopter of AI. Can you explain to everyone what you all do at Podetize and then take us back to the early days of you all using AI?
Podetize is podcast and monetization put together. Our platform was always built with the technology of being able to mix your own promotions and ads and other things to make sure that you could monetize your business-to-business podcast. It’s our focus. We’re not really focused on the entertainment style shows. We’re focused on the independent business-tobusiness use of podcasting. So think of it like content marketing, and that’s where our specialty lies. Initially, we started out offering all these production services.
We knew we would eventually want to offer this sort of, I’m going to call it do-it-yourself way, the way to use the technology to do it. But the technology wasn’t there yet. The AI hadn’t caught up yet. There was just pieces and parts that were starting to come in, but they weren’t really there and they required too much training and my clients were never going to do that. They don’t want to sit through a course, they just need it done for them.
So we developed these done-for-you services, but with the intention that we would learn from that and start incorporating things into our self-service model as fast as we could. That was the intention over time. We always started with video, audio, blog and social share. But video went from like 10% of the users to 60% over the years.
And social share, we don’t actually go into people’s social media and share for them, but we provide them what they need to share out there. So that’s kind of our model of it. But in doing so, we are touching so many different aspects of their business. And everything has to flow through, because as you mentioned, YouTube today, YouTube’s owned by Google. It’s an SEO strategy, it’s a search engine optimization model. Everything we do has to be titled right has to be captioned right, has to be described right. Everything in that process. And by controlling all the pieces for our clients, we started to understand the continuity across all those pieces. And that’s really where we started to find, Okay, things are really working here.
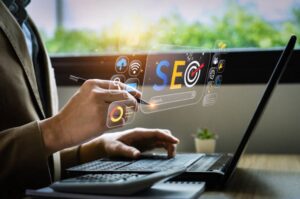
Thinking of those early days, who did you have on your team?
One of the very first things we did was have a transcription team, and they were fantastic because we needed humans, because the AI was so bad transcribing in the early days. And so we actually put AI as our first layer, but we would edit it basically four times on top of that in the early days. And then the transcriptionist would go through and clean up all what they called unintelligibles, the things that the AI couldn’t interpret, which was a lot back then. It was probably about 40% of it was unintelligible.
It’s gotten better over the years, but it’s still somewhere between 70% to 80%, depending on the speed of the speaker. I talk really fast, so it’s probably more like 70% on me. Then we would go through it and fix industry terms, things that were misunderstood. I worked in the e-commerce area for a while, you would have SKU, which was stockkeeping unit. It was an acronym, but very often the AI would interpret it “skew”. And you would have to go in and clean those things up, so you would have to know something about the industry that you were working with. And it would take some time for our transcriptionists to get up to the level where they could
understand, and we started to having to group them together. this started to give us this idea that in order for the AI to get better, it needed to learn an industry lingo.
That naturally begs the question…how do you teach the AI an industry lingo?
Well, there really wasn’t a way to plug in some dictionary. There really wasn’t a way to do that because everybody used them differently. They sometimes have different meaning. They’re usually emerging in an industry, especially something like “real estate,” when they’re talking about distressed notes and various weird things, that there are actual definitions for those words, but they don’t mean what you think they mean. And in real estate, they mean something completely different, right? So that’s what we started to learn of it.
And then everyone has their own sort of way of speaking and way of using terminology. And honestly, there’s generational speak that scares the heck out of me. I happen to have three daughters who are all from a different generation, so I have a Millennial, a Gen Z and a Gen Y. It’s crazy. And they all have different words that they use, and they mean different things. So this is where we start to get into that, how do we do this?
What we found was the AI wasn’t getting any better. Over time, it was getting worse. And so we were like, What’s going on here? We’re doing all this work, transcribing it, we’re getting it in the blogs, we’re doing all of these things that we’re supposed to do, but the AI is not getting better. Shouldn’t it be getting better? And what we realized is that it’s built without the feedback loop. It doesn’t know when you pop it into some AI transcription thing, it hands you back a document. Then you take that document and you go do what you’re going to do with it. Write an article about it, put it in a blog, make show notes for your podcast.
Whatever you’re going to do with it, you do that separately. You never send it back and said, “You screwed up on all these words,” so it doesn’t have a feedback loop to learn how it was. You might in some transcription services say, How accurate was this? And you could give it a rating. But that doesn’t teach it what it missed. And that’s really what we started to refine. Internally, we created the feedback loop. That’s why we actually incorporate our AI into our platform. In 2018, we only used it behind the scenes, so only our team used it. The front facing clients did not. In the next 30 days, they actually will be able to use it on the front facing.
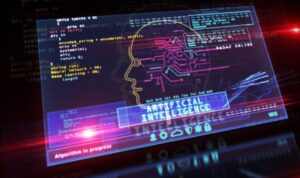
That’s amazing Tracy! And the fact that you all started this back in 2018 is even more amazing.
So anyone who has a podcast can go use our AI transcription system. But for right now, what happened was that we would feedback what it was, and so then we would teach the document to compare. Have you ever done that in Microsoft Word compare docs, Google Docs, compare to the final redline edited version, and where are all the changes? And so we would teach it to compare itself, and then it would learn where it went wrong, because we would say, “This one we did is final. So this one’s more important.”
I don’t know about anyone else, but this is particularly interesting (and relevant) to me because we use an AI transcription tool. Once we upload an audio file, this AI tool provides a transcript within 10 minutes, but then we do additional editing. How would we send these edits back to the AI tool that we’re using?
See, you can’t? All those tools were built not to do that. And most of the tools were built so that you would get your output and you just take it with you. So you were not actually using the tool to publish, but our system, you publish from it. We have the ability to edit the transcript right there, or drop the corrected one into it and teach it to compare the two and learn patterns. We wrote code that would teach it, When we drop this final in, your job is to compare it to what you originally transcribed, AI, keep a cheat sheet of key learnings of what you learn from doing this.
And then we started to say, Okay, now I want you to make key learnings about the industry. So we’d take all, like 10,000 episodes from the real estate market, and we’d say, I want you to make key learnings about the industry lingo. So we would teach it to learn about the industry terms, it doesn’t understand women as easily as it understands men. The accuracy on transcription just isn’t really great between men and women. It’s actually about a 15% differential.
Wow!
And that’s not good. But over time, ours equalized without us having to do that, because we happen to have an equal number of men and women. So the podcast industry in general, and the transcription industry, if you think about dictation, is very male dominated, and that’s why there’s such a discrepancy there. It’s just not used to hearing women’s voices. But our system equalized actually really quickly without us having to intervene. But what if we had a lot of Spanish speaking podcasters all of a sudden, and there was a dialect, there was an accent, we could do a training set to help it learn that and create a pattern of how the word sound. So what
you should transcribe instead? And we would have a better opportunity for doing that than most people would.
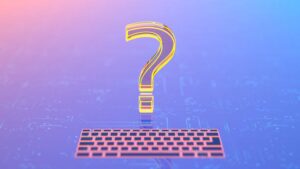
It sounds like Podetize has created its own transcription AI with a feedback mechanism. Is that a fair statement?
Yeah, I mean, look, we built it on top of another system, but it’s interchangeable. So originally we used one AI system. We tried OpenAI, we didn’t like it, we switched to what Bard is based on. I think it’s called Gemini now, but it’s PaLM, if you want to call it that. And that’s Google’s version of it. Google’s version is better for what we found. It has a higher accuracy level at stage one, even before we put our training set in. And then it learned really quickly when we gave it the training set.
So this is kind of, its progress matters, but it basically means that tomorrow, if Bard outdoes PaLM, we just can switch it out so we can be agnostic to the large language model that it’s built on. You know, in this market, it is risky. What if a company goes belly up and you’ve built everything customized on it? So we built it so you could pull that piece out and our piece would still exist and all the learnings that we had it create would exist, and the new AI would just learn that.
Tracy, when we were backstage talking, you mentioned that there are three things that a good AI tool should have. One of those things is a large language model. When some of us hear those words, it sounds complicated and scary.
Yeah. Large language model is like what everything is built on. It’s all the things that OpenAI went out to scrape and pull and get data in, and it needs a constant fill of data and information at all times. So, it’s basically all the encyclopedia library of things that it’s been learning from over time. In the case of OpenAI, it was mainly built on the spoken word, the written word, like all of those things that built all kinds of things in there. They used audiobooks, they used Twitter, they used Reddit. They wanted it to be conversational.
In that process, they went for basically a large language model that was built a lot on conversations. PaLM and Google’s version of Gemini are built more on all the things off the Internet, the written word blogs and our websites and all the things that are out there that are written. So it has a different model that is maybe, I think personally in my use of it, is stronger in search engine optimized results. If I want to write an article, if I want to title something that’s going to be a blog, a podcast episode, I probably want to use that because it’s farther along, it’s learned from a lot more data.
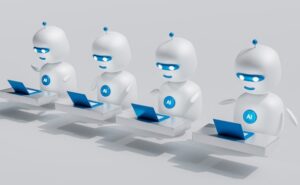
I think some estimates are it’s got like three to five times more data than OpenAI’s large language model is based on. So it’s more information and it fills at a faster pace. When you think about that, that’s really important, too, because we could get diminishing returns from our large language model if it’s not learning new things fast enough, meaning it’ll kind of flatline in what it can do if we don’t give it a lot of new information because as I pointed out, my younger generation comes up with new words every day.
If you’re not building that in, you’re not learning from it, you’re not growing. We’ll get a lot of redundant and similar results. So everything will be homogenized, and we don’t want to be that. We want real, original, continued thought leadership. The large language model becomes that really critical growth piece, and I want to be able to switch it out to whoever’s best at whatever the end result I want. So if I want to use it for my customer service, I probably want to use OpenAI because I want better conversations.
Okay, so OpenAI for conversations and PaLM for research-based written communication. By the way, for those of you that may not be aware PaLM is the AI large language model developed by Google. That’s what Gemini is built off of – remember, that’s the tool that Dr. Terica Pearson spoke about in the last AI demo session. What about images, Tracy? What is the preferred AI large language model for images?
So I’m thinking about where I want to use it if I want imagery. There’s a couple of new players in this marketplace in research right now, and there’s some new players out there, and I’m very eager, and we’re going to be working on a customized solution out there. I’m always looking for this because I don’t think the player is going to be with what we’ve seen already in the image world, I don’t think it’s good enough yet, and that’s because I come from a design background. I’m seeing some promise out there, but it’s going to be a totally different language model.
If we can take a quick detour – once you select a large language model that you want to build an AI tool on top of, do you then feed it an initial data set to that it can learn things? And then, moving forward, you add more data so that your tool can get better at generating results?
So it’s a combination of the training data set and prompting. You guys have heard prompting, I’m sure, before by now, in the whole AI process, prompting is what you ask it to do, right? How you’re instructing it to do it. Training is like, here’s this other library. I want you to compare and contrast with that, but here’s the prompt. Here’s what I want you to do with it, how I want you to use it. And those things go hand in hand with creating a really rich training data set, but also customizing a solution that’s going to optimize your business use case. So in our case, we want it to be, if you want to title your episodes right within our portal, then we’re going to be
teaching it. Here’s 50,000 episodes that we personally titled that we know rank on Google, that we know rank high in the search engine already. Now go one step further and learn from Alicia and make sure you title it in a way that she would approve. So it has her brand styling in it.
And it’s in my voice.
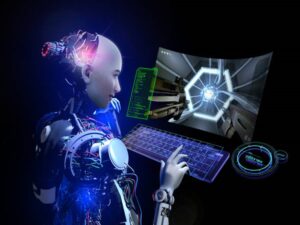
What we found is somewhere between 20 to 50 episodes. That’s all it takes, 20 to 50. It depends on kind of your model. If you have a lot of guests, it takes longer. But if you’re doing a lot of subject matter episodes, then it’s easier, it can go faster. And in that model, it will learn very quickly your brand, and it will get it right about 90% of the time. The first time, it generates a result for you.
And that’s important for it to get it as close to being right the first time around as possible. It saves us even more time in making corrections.
Because you’ll get frustrated right? If you had to generate, like, ten every time.
Exactly!
Okay, well, back when I wrote for Inc magazine, they used to make us submit ten titles. Because people are so bad at titling now, I could do three, and I’ve got one that I know is good.
Okay, back to my previous question about three things that a good AI tool should have. Again, you’ve mentioned a large language model as one, but what are the other two?
So it’s that training and prompting set combined to really customize what you want to do with it and making sure that it has a continual process to grow and learn so that it’s not just taking the large language model itself, it’s taking that and creating proprietary learnings on top of it, things that you know in your business. So think about it from this perspective – whatever it is that your staff and your team are so good at learning, and then you lose that employee and you have all these key learnings that you just lost, and you have to start all over again.
It’s hard to teach that. That’s what we want the AI to always have and should be proprietary to you and your business. It should be in your training data set, in your prompting. Those things should be core to your business, and it will make whatever you use more valuable for you. So think of it like your proprietary process.
And you know we’re all about processes on this show, Tracy! In fact, processes are one of the three elements of business infrastructure. As a reminder everyone, business infrastructure is a system for linking your people, processes, and tools so that you can scale your business in a repeatable, sustainable and profitable way.
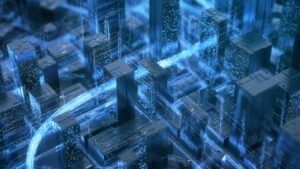
With that in mind, if I understand you correctly Tracy, there are certain things that people on your team may be doing. And if they were to leave, that knowledge basically leaves with them, unless it is documented somewhere. But with an AI tool, you have the ability to store that information, add to it, continuously refine it, and then it can do some prediction, not just the generation of information based on prompts or commands that you give it.
Yeah. So think about it like this. I might have a top copywriter who had previously titled episodes, and she was just better at it than everybody else. She was just really good at getting the message, getting the voice of the podcaster in the title. I can send that through and say, I want you to model my top ten salespeople, my top ten copywriters. I want you to model what they do and figure out what their process is, what their learnings are, what they’re basing this on, learn how to do this, and it will.
That’s how smart it is nowadays. AI has come so far in the last five years just on that piece alone. That’s where I got really excited about it when I saw what it could do. Because prior to that, it wasn’t learning anything from its past. It was heading into a place where, you know, how have you ever had an assistant? And if you tell them to do something one way, that’s the only way they will do it from that point forward, because they’re so afraid of disappointing you?
Yes.
That’s what AI was like before. It was like, This is the path of least resistance. If I give her this, it’ll be fine. And so what happens is that everything sounds generic by the time you’re done with it. There’s no originality, there’s no energy to it anymore. And so that’s the difference between you giving it this customization and forcing it to continually up its game.
I like that – up its game. Okay, that covers the second thing a good AI tool should have. The third thing is the feedback loop, right?
Right. Because, look, you’re nothing if your customers don’t love it, right? I mean, at the end of the day, I can think, I’m doing the best job here. I’m training and I’m learning. But if it’s not acceptable to my customers or the market changes, and it’s not acceptable to YouTube anymore, it’s not working. So we have to have a feedback loop for that. But the problem is that it’s thumbs up or thumbs down and ask you to comment on it, and you have to describe that.
And people aren’t good at that, and they don’t have the time for that. So creating ways to passively judge whether or not they’re doing this is one of the ways. We would do this in the early days. We would take our transcripts and we would run them and then drop them in. It automatically knows it didn’t get it right. You don’t have to say you did this wrong. It just automatically knows by comparing it.
So if you put a comparison process, a feedback loop into it that says that you rejected this, then you know you have a problem. In our titling, which is behind the scenes, you can’t use this on the front end, but in the next six months you will be able to, but you only get one recommendation. And we did that on purpose so that as you drop in your podcast, audio and video and it generates a title recommendation based on your history, based on our best practices, based on our length that we recommend because you’re going to use it on YouTube.
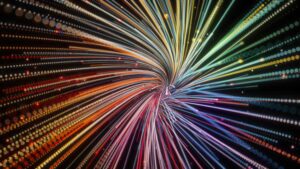
So it needs to not be longer than 70 characters. We’ve optimized it already for you so you don’t have to do as much thinking or work about it. And it pops up a title and you say, “Yeah, I don’t love that one. Let me click refresh.” So you click the little circle, that redo that we’re very used to when we use Chat GPT and anything else we regenerate. So you regenerate the next one. Now, if the next one’s right, you just hit submit and it’s done. Now you’ve accepted it. Now it’s going to compare the last one that it tried to, this one, and what was different. You don’t have to tell it what was different. It’s going to learn what’s different.
And then if you go multiple times after the third or fourth time, it’ll ask you why you’re regenerating. What am I not doing right? What do you want me to learn? Because it needs an input at that point. And it’s going to prompt you with ideas like more positive, higher energy. It’ll prompt you with common things that you say. So we used to get this a lot in our graphics. People don’t have a visual language. They can’t speak design. And so they can’t say, “I wish it were more sunny”.
You can be critical about it, like, “I don’t want any human beings in my images”. But if you’re not that specific about it, people don’t know what they did wrong. The designer doesn’t know how to fix this. And that would happen really often with my graphics team. And they would be like, I don’t understand what’s wrong with this. So we actually created a library at the beginning when we would onboard a client and we would create a visual library of the types of images they felt satisfied with.
And then we’d create a learning and we would have our head graphic designer would go through and create some visual language learnings. Oh, they hate the color orange. They couldn’t even articulate that. But we would know because everything we gave them they would reject until they got to this little dozen image set of what they found acceptable, and then we would make all the learnings from it, and so we would do this manually. Now we can do it automatically.
Tracy, you know what this reminds me of? Years ago someone told me, “It’s easier for most of us to say what we don’t want than it is to say what we do want.” This is a good segue to another obvious question – how can you build an AI tool for your small business? Who can build it for you? Do you have any recommendations?
The first thing that I do recommend is that you need to make sure that you intimately understand how AI works in general. Go use it! And I find too often that the heads of companies have never even popped onto Chat GPT and tried it. You must try it. And then what I
recommend people do is that whatever you did over in Chat GPT, go do it in Google Bard. Just try multiple platforms, try different tools, see what they’re like, and you do the same thing twice.
This is how I taught every member of my team to use the tools. I actually had a class. I did it for all my clients, and I call it my AI 101 kind of class. Iit teaches the basics of how the power of your questions, the vocabulary you use when you’re asking it things, adjectives, verbs, how you’re getting it to do what you want it to do, is extremely important. When we treat it like this tool that should just know everything, it’s a mistake. It’s like assuming that our team knows what we want. It’s mind reading, and that does not exist. We cannot get good team if we don’t train them, if we don’t support them, if we don’t give them feedback and let them know what they’re doing right and what they’re doing wrong. It’s the same thing. AI is a team member, so you should get to know it, just like you get to know every member of your team and understand just the basic fundamentals of it.
The next step is that you do need to accept that this is an experiment, that there are a lot of people out there who claim, “Oh, I can program AI”, but they don’t know what they’re talking about yet. They’re all learning on you, they’re learning on your dime. So you have to just be willing to accept that and say, “Hey, I’m investing in you to work with me and let’s do this together. You’re going to learn a lot about AI and you’re going to learn a lot about programming and things are going to go wrong, and that’s okay. But we’re going to be in this noble experiment together and we’re going to get something out that we didn’t even imagine when we started our plan for it”.
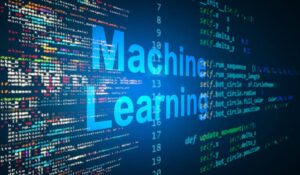
Should we look for programmers that specifically that have a specialty in AI? Is there a certain title that we would look for?
I like to work with coders who are always willing to learn the new thing. They’re not stuck in their ways of the thing that they’ve always used. So if they say, “Oh, I only code in this language”, whatever it might be, I’d be like, “No, you’re not my team member, right? I want you out there experimenting.”
I made the poor guy who built my portal, do a tiny little couple hundred dollar project to put that AI transcription system in an automation into my Dropbox folder so that I could link it from ClickUp and Dropbox. That’s all I needed was to create the conduit between this API for the AI. He just did such a fabulous job of thinking things through for me.
And he said,”You know, I think we should build it this way because it’s going to have more longevity, less will break in the future. You won’t have to repair the automations as often. We’re not going to use a zapier, we’re going to do it this way.” And he coded all this stuff and I got major money’s worth out of that because he just made my whole system work faster. Someone with that kind of structural thinking is going to be much more valuable to you here than someone who can program, and that’s hard to find. I think you have to have some comfort level with them and also just some grace, because it’s not going to work out every time you try something, it’s just not going to.
Like you said, and just keeping in mind that it’s experimental, right?
Yeah. And that’s why we put everything behind the scenes and try it out with our team, because if our team gets frustrated with it and refuses to use it, it’s bad. We need to make repairs. But if our team is like, This is the best thing. Could you do more? Can you automate this more? Now we know we’re onto something, we’ve made their life better.
Let’s talk a little more about your company’s AI transcription tool with the feedback loop. Is it available to anyone or only your clients?
No, you have to use it from within our portal. However, it is in our long-term business plan to be able to create APIs for other companies to be able to utilize it in their systems, in their processes. So in a sense, we created not only something that we’ll use intimately in our portal, but we can license it out to other companies that might be doing videos, that might be doing high levels of blogging or other things that might come from the spoken word or audiobooks. Wouldn’t it be great if you just had the audiobook version and then you had the written version? What if it could do that?
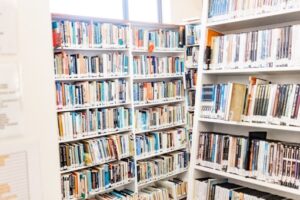
That would be great! As we near the end of this fireside chat Tracy, can you share some resources where we can learn more about AI and how to build our own AI tools to improve our operational efficiency?
I am a big fan of getting into a community that is experimenting. This is where our coders have a lot of advantage. They’re in Reddit groups and they’re in coding communities and they’re all chitchatting back and forth and they’re progressing at a faster pace because they’re being open about it. It’s why OpenAI was built the way that it was, right? They’re being open about it.
Hence the name, Open AI. You mentioned a class you’ve taught your team. Is that available publicly?
It’s like ten part class, which I am always happy to share with anyone, which I was just showing my clients how to play, how to experiment.
It’s in Zoom. It’ll be there for because I promised everybody it would stay in Zoom for a year. So it’ll be there for a while and you can go and play with that and just learn from that. I also have a very. It’s called AI For All. It’s a circle group. If you’ve ever done circle.so, aiforall.circle.so, and a good friend of mine, Joy, runs it. And what I really love about her is she’s always experimenting.
She’ll just show you how she built an AI bot, she’ll share it with the community. And everyone within the community does that. They just gift their courses and gift their conversations, and they’re all so rich about it, and they’re anxious for everyone to grow together and collaborate. And this is a really great community. Plus Joy and I really believe in the “ethical use of AI,” but I don’t think that’s really the right term. I think we need to make AI for social good, be its model.
In other words, look, I’m going to eliminate jobs like it’s going to happen. I’m going to decrease the amount of transcriptionists I need because my AI is getting better and better at it. But I’m personally committed to retraining every one of my team members. And right now that’s actually what we’re doing. We are training all of our transcriptionists to be bloggers because blogging is custom. Like, you have to format everything, and it’s a great opportunity for them to copy edit and double check and make sure the AI didn’t mess up. And we still didn’t miss anything in the process.
But now they’re becoming 90% blogging, 10% transcriptionist. So their job is a little more enriching, it’s less mundane. So if I can improve their lives and give them a career opportunity that’s higher paying for them, then I have done some social good. Even though I eliminated a job type. I think graphic designers are in danger, and they’re the ones that I’m most concerned about. How are we going to be able to give them a role where the creativity. And so I have made it a mandate within my company that every graphic designer who works with me must use a tool that has an AI function in it, and must try AI with everything that they do, so that they’re learning it as a part of the process. And then they can see where is this going to benefit my job, so that they can figure out where AI fits in to supplement their work.
And that way it isn’t something that happens to them. They have been learning it all along and have some time to think about how it can be used in the future.
Right? And they’re going to think of a great way to use it in a way that I would have never come up with because I don’t do graphic design every day. They’re going to think of a great way to serve our customers to get the end result that it’s going to be amazing and make their job easier and make it so that they can spend more time on the creative function.
Tracy, you are such an inspiration. I love the energy, enthusiasm, and passion that you always show up with. How can people find you online and connect with you?
Well, I am on LinkedIn, so please follow me there. You can find me, Tracy Hazzard with two z’s. And we actually do a live stream every week about podcasting to our clients and to the broader podcasting community. We also stream that out on YouTube as well, so you can follow Podetize on YouTube. And we just really want to make sure that we’re having conversations out there. So the more you comment back, the more you give us feedback, the more we can tailor things for what you’re really interested in discussing and talking about. And we do hit on AI a lot in our conversations about how to use it. And sometimes I’ll demo new tools on occasion, but most
often it’s just an experiment that I’m sharing with our community that we’ve been testing. It’s not really a rollout.
Tracy, thank you! It’s always a pleasure speaking with you!
You too. Absolutely love geeking out on this with you!
Let’s give a warm round of applause for Tracy Hazzard!
In case you’re wondering, Tracy and her team at Podetize don’t have a name for their tool yet, but rest assured…they’re working on it. She mentioned several resources throughout this fireside chat, all of which you can access links to at BusinessInfrastructure.TV. Again, that’s BusinessInfrastructure.TV.
This concludes our AI Audio Conference. I’ll share my closing remarks in the next episode and tell you about another exciting season we have coming up.
Thank you for listening! If you enjoyed this episode, then please subscribe and give us a five-star rating and review.
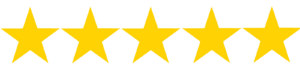
Until then, remember to stay focused and be encouraged. This entrepreneurial journey is a marathon and not a sprint.
This episode was produced by me, Alicia Butler Pierre. Audio editing by Olanrewaju Adeyemo. Voiceover by Kenya A. Moses. Original score and sound design by Sabor! Music Enterprises. Video editing by Gladiola Films. A special thank you to Grant Revilla for creating the show notes.
This is the Business Infrastructure – Curing Back-Office Blues podcast.